Use Case
Mining Industry
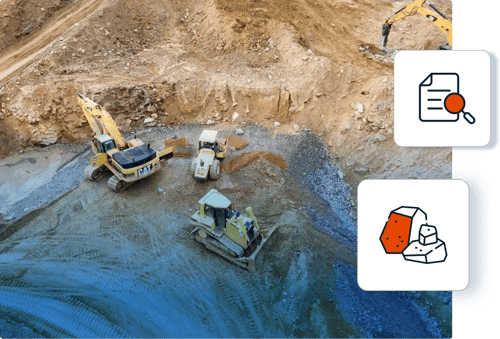
Industry/ Mining
Functionalities
#machine-learning #data-analysis #data-optimization
Mining operations yield increase through digitization
The Mining division of an international Steel producer wants to explore why the yield (in t/h) of some production lines is different from other identically equipped lines. Yield can be improved through analyzing data such as root cause and material specifics.
Challenge
Yield optimization, previously done by non-digital means, will now pass into the hands of Wizata’s data-oriented machine learning model platform. The goal is to prove that digitalization is superior to conventional means by improving ROI.
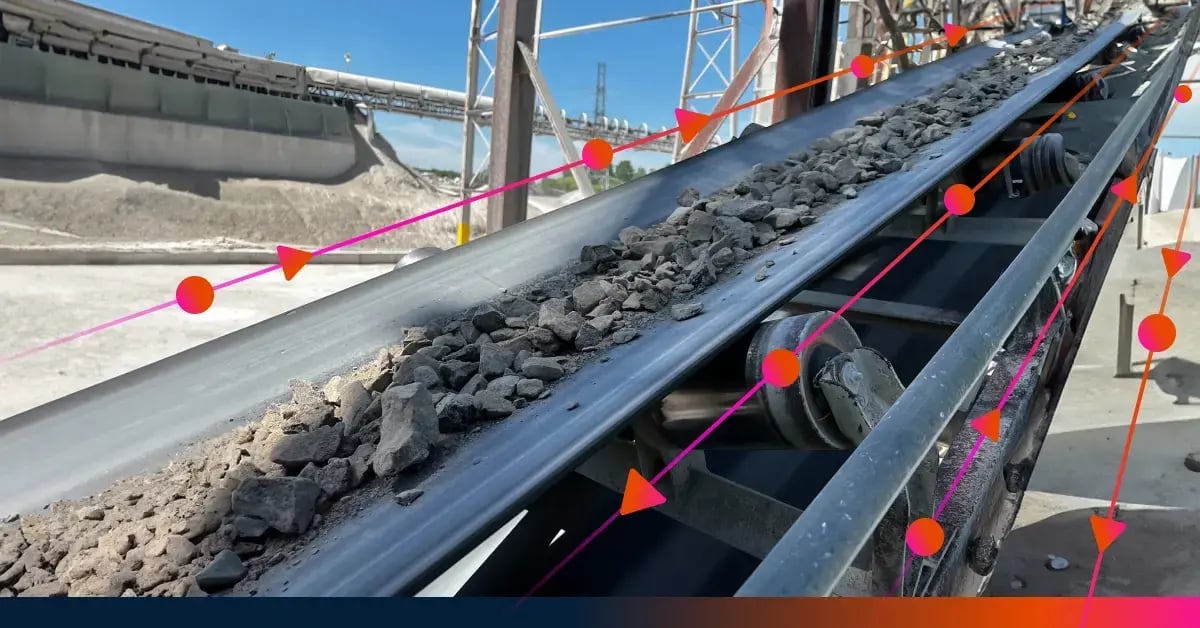
Approach
The first step is the importation of 73 years of historical data. This data shall then be cleaned, transformed, and formatted to enable analysis, reporting, and machine learning modeling. An Al platform core algorithm is used to re-align the data to enable a better understanding of how different components like power and water usage and grade of contamination impact the production process.
Aligning the data with the business processes and advanced analytic techniques reveals that the features with the highest impact on the production rate are:
➜ Mill feed – the amount of material going into the silo
➜ Sifting screen – the speed of the sifting based on the desired separation of the material
➜ Amount of water in the R spiral – based on how the material is crushed, water needs to be adjusted
Statistical and machine learning techniques are used to recommend optimum settings and actions to steer yield in real-time and generate revenue.
Outcome
An Al-driven recommendation system has been deployed on six lines to help operators to optimize the yield in real-time through optimal setpoints guidance. The yield was increased on average by 1.5% per line per year. ROI on the aforementioned lines surpasses a yearly increase of $2 million per line.
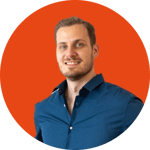
Get in touch with one of our experts
Filip Popov